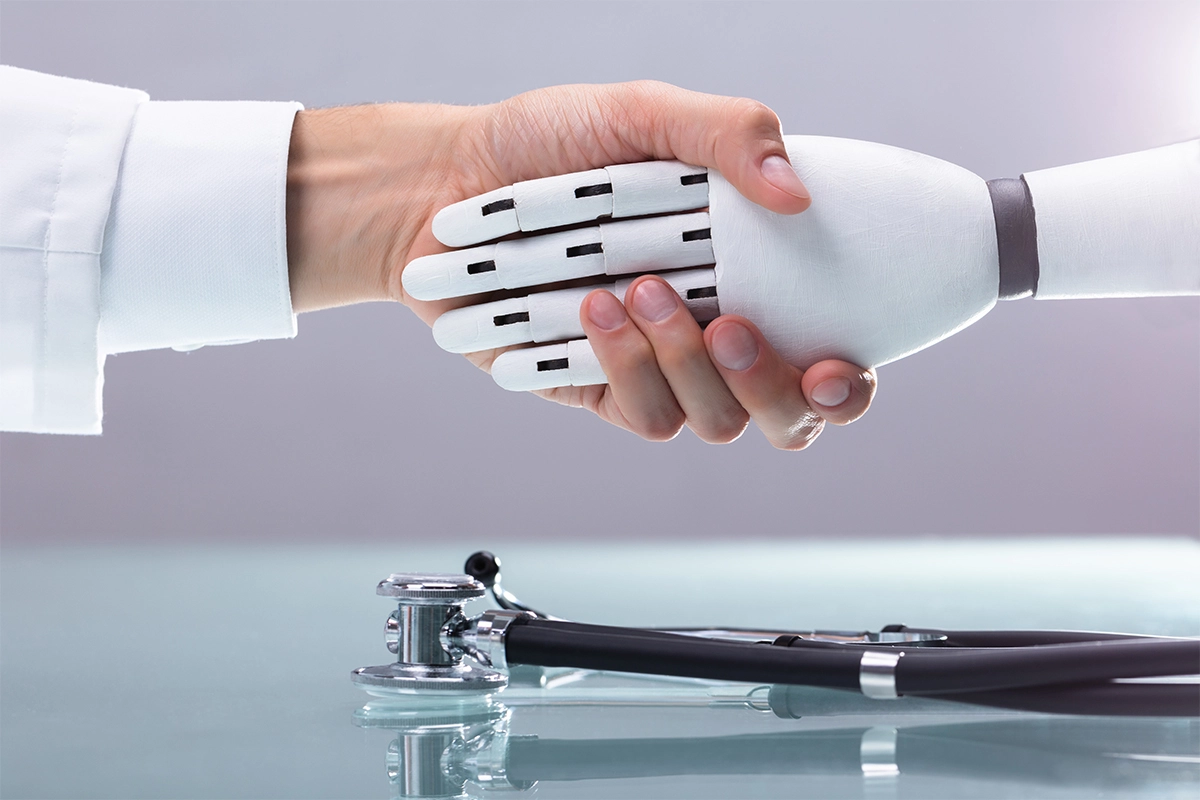
Generative AI continues to spark significant interest and discussion, especially within the healthcare industry.
However, it's important to note that generative AI alone, while pivotal, is not a panacea – its applications are nuanced and varied, and there's no one-size-fits-all approach to its implementation.
The field of AI encompasses diverse models and methodologies, each with advantages and drawbacks. The decision as to which AI models to rely on requires careful consideration, as patient needs are multifaceted and evolving.
Health system leaders need to understand the nuances of generative AI and other models in patient-facing care, so let’s dive in.
What are the kinds of A.I. models to even consider?
The variations in AI models and methods are crucial factors that contribute to the success of patient-facing applications. Different patient intents necessitate different AI models to ensure the highest levels of trust, accuracy, and actionable insights. The diverse range of clinical, administrative, and informational intents requires a balanced mix of AI models to deliver desired and comprehensive outcomes.
So what are the options among AI models to consider? We’ve laid out the following basic framework to help healthcare organization leadership get a sense of the pros and cons of varying AI approaches. The right AI model to use depends on the patient’s intent, with the goal of providing the most accurate and robust information for every intent.

Striking the Right Balance: The Role of Expert Systems and Generative AI
Referencing the diagram above, consider highly clinical triage use cases. In scenarios where patient health is at stake, expert systems take precedence. Medical best practices and wisdom have evolved over thousands of years, incorporating validated clinical logic. For example, determining the appropriate care based on symptoms, urgency, and medical history demands unwavering accuracy.
Expert systems, exemplified by nurse triage protocols pioneered by Dr. Barton Schmitt, provide gold-standard algorithms for routing patients to the proper care. These protocols have withstood the test of time and are backed by years of real-world usage. When patient safety is paramount, relying on well-established expert systems is non-negotiable.
On the other hand, administrative tasks and web navigation, for example, are more suited to generative AI models. Scalability is a critical factor in these use cases, where the abundance of data input allows generative AI to provide highly personalized, robust responses with high sensitivity (i.e. few false negative results for the input). Automating administrative healthcare call center interactions and assisting users in navigating complex websites benefit from generative AI's flexibility and adaptability.
Understanding Patient Journeys and Conversational AI Use-Cases
Our experience deploying patient-facing AI for complex clinical scenarios in health systems across 15 states provides us invaluable insights. We understand the importance of data sets, training methods, and integration strategies for different patient intents. This knowledge forms the backbone of our framework, ensuring that a suitable model is employed for specific patient intents.
Before diving into the details of our intent-to-AI-model framework, let’s consider the following broad patient-journey construct. By looking at a patient journey in the following way, we can see where opportunities for conversational AI come into play.

The essence of the above patient journey diagram is:
- When a patient is trying to interface with their provider or health system, their intent and purpose should easily and accurately be understood (i.e. natural language processing via both voice and digital)
- Once their intent is understood, a robust NLP should trigger the correct conversational AI model that asks the right follow up questions to maximize accuracy and actionability of the patient encounter
The Power of a Hybrid Approach: Our Unique Framework
Leveraging our framework, our vision is to create the most clinically robust, trusted, and actionable personal healthcare assistant that revolutionizes the patient-consumer experience. This vision holds substantial benefits for health systems and payers alike, driving revenue growth, cost efficiency, and patient satisfaction.
To address the diverse range of patient intents, Clearstep has developed a unique AI framework. By harnessing the strengths of expert systems, neural networks, and generative AI, we're crafting a comprehensive solution that caters to the varied needs of patients. Below is an evolving yet fairly comprehensive look at how Clearstep is developing models for the widest set of patient intents and use cases, ensuring the highest degree of accuracy for all intents.
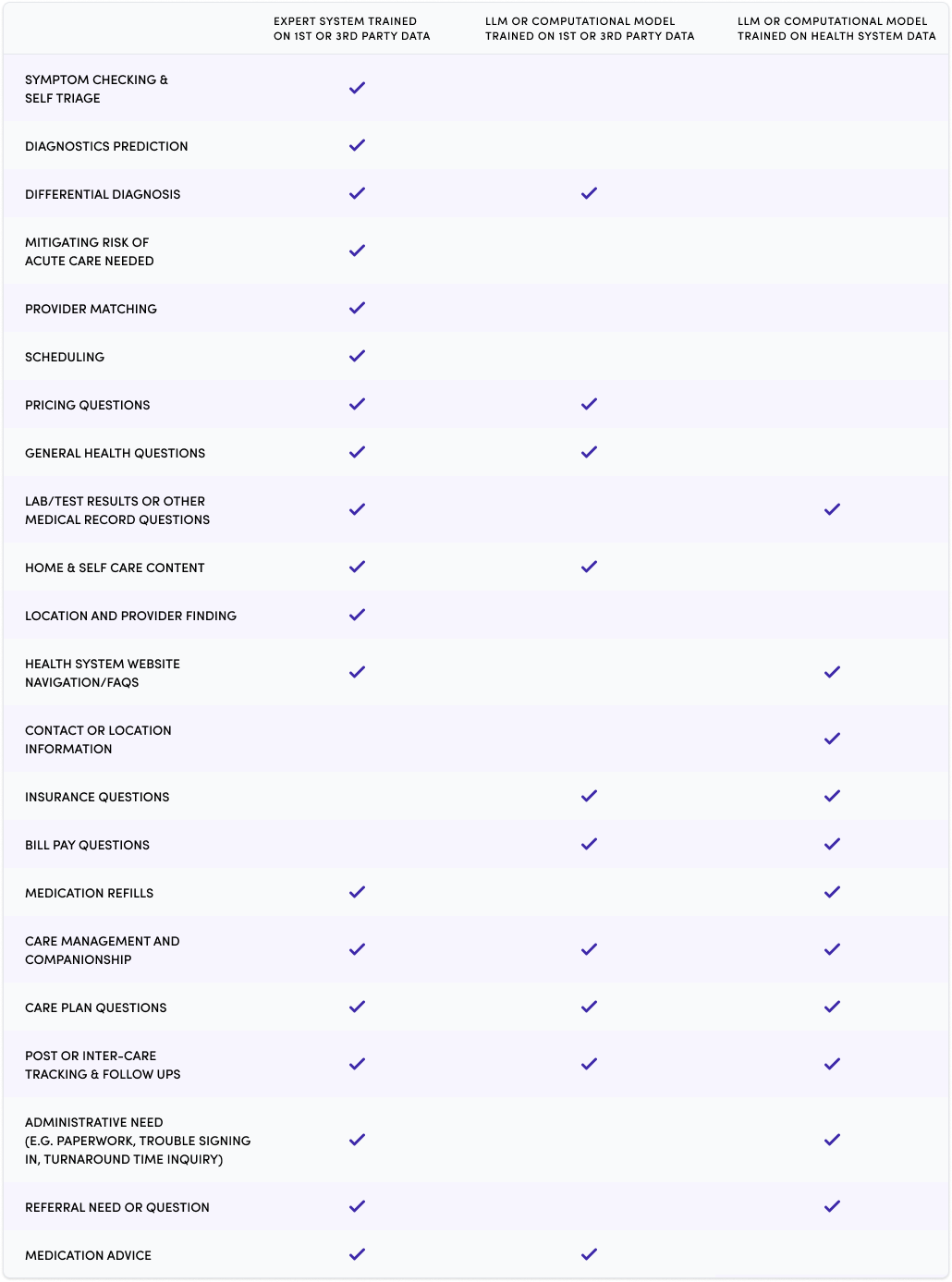
Embracing the Future: A Data-Driven Patient-Centric Approach
Our AI framework paves the way for a new era of patient-consumer interactions, elevating care quality and patient engagement. By mapping patient intents to suitable AI models, we ensure that exchanges are automated, highly personalized, and accurate.
We acknowledge that not all patient needs can be met with a single solution, and our hybrid approach allows us to offer the best of both worlds to maximize the potential of AI while ensuring patient safety and satisfaction.
Interested in what our digital triage technology could do for your organization? Let's talk.