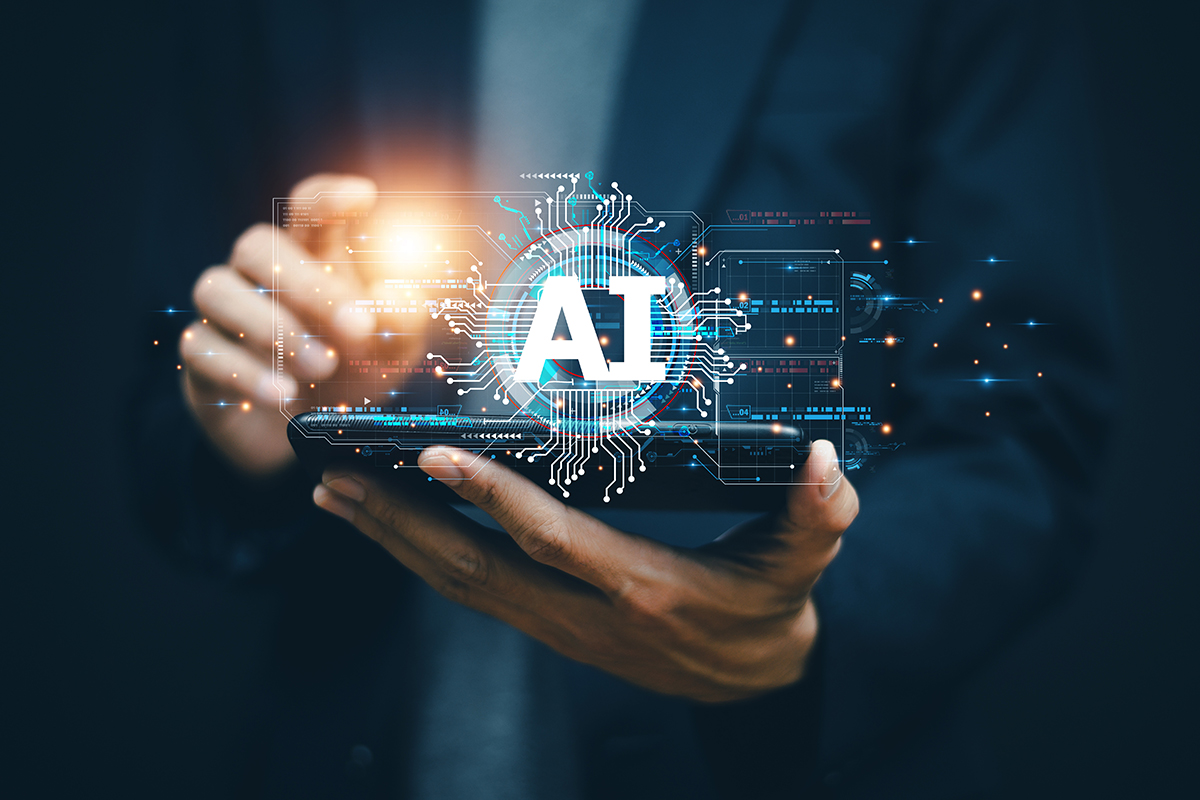
Artificial intelligence (AI) is now deeply entrenched in the healthcare industry, driving significant advancements in how patient care is delivered, managed, and experienced.
The integration of AI technologies across various facets of healthcare is reshaping the sector, promising improved patient outcomes, enhanced operational efficiency, and innovative approaches to medical challenges.
Let's explore several key trends and applications that characterize this transformation.
Transformative Technologies in Healthcare
While often wielded as a broad brush term, AI encompasses and works with various technologies, each offering unique capabilities and applications.
Key healthcare technologies include robotic process automation (RPA), natural language processing (NLP), machine learning (ML), and generative AI. These are progressively transforming healthcare by automating routine tasks, enhancing decision-making, and facilitating new medical advancements.
Be sure to check out this article for a helpful breakdown of AI automation’s five levels.
Robotic Process Automation (RPA)
RPA involves using software robots to automate repetitive, rule-based tasks.
RPA is widely used in healthcare to streamline administrative processes, such as data entry, patient scheduling, and claims processing.
For instance, healthcare organizations employ RPA to automatically extract and verify patient information, reducing administrative burdens and minimizing human error. This automation enhances efficiency and frees healthcare professionals to focus on more complex and value-added activities.
Natural Language Processing (NLP)
NLP enables the extraction of meaningful information from unstructured data, such as doctor's notes and discharge summaries.
By converting text-based information into structured data, NLP facilitates better data analysis and decision-making. For example, our NLP conversational AI assistant matches patients to the appropriate workflow for services requested.
Also, NLP aids in categorizing and analyzing clinical notes, enhancing the accuracy and accessibility of patient information.
Machine Learning (ML)
ML algorithms learn from vast amounts of data, identifying patterns and making decisions with minimal human intervention. For example, our large language models (LLMs) use ML to predict the probability of a given word sequence within a particular context.
ML is particularly valuable in healthcare for predictive analytics. It can predict potential health issues by analyzing real-time patient health metrics, allowing for timely interventions and improved patient monitoring.
This predictive capability extends to operational areas, such as forecasting patient volumes and optimizing resource allocation, thereby enhancing the overall efficiency of healthcare delivery.
Generative AI
Generative AI, which includes large language models, can create new content, ideas, or data.
GenAI has numerous healthcare applications—from improving clinical decision support to accelerating drug discovery and development.
For instance, AI can generate detailed patient reports and facilitate sophisticated virtual triage assistants, providing comprehensive support to healthcare professionals and patients. However, the deployment of generative AI must be handled cautiously to mitigate risks, such as the generation of unreliable information.
Achieving Interoperability with AI
One of the longstanding challenges in healthcare is achieving interoperability—the seamless sharing and utilization of information across various systems.
AI technologies are pivotal in addressing this challenge. By standardizing diverse data formats, improving data quality, and automating data sharing, AI facilitates the efficient exchange of information across healthcare systems. This ensures that healthcare professionals can access comprehensive and accurate patient data, enhancing clinical decision-making and patient outcomes.
AI-driven interoperability also enhances data security and compliance. AI tools monitor data transactions to safeguard against security breaches and ensure adherence to healthcare regulations. Additionally, AI enables real-time data analysis, providing healthcare professionals with immediate, actionable insights crucial for timely and effective patient care.
Strategic Implementation of AI
The successful implementation of AI in healthcare requires strategic planning and consideration of several factors.
Healthcare organizations must define clear objectives for AI adoption, whether to improve patient outcomes, reduce costs, or enhance operational efficiency. Assessing data readiness—including quality, quantity, and accessibility—is also critical for the success of AI initiatives.
Additionally, ethical considerations must be addressed to ensure responsible AI deployment. This includes obtaining patient consent and addressing potential biases in AI algorithms.
Organizations must also decide whether to build in-house expertise or collaborate with external partners to implement AI solutions effectively. Choosing scalable and integrative AI technologies that align with the organization's needs is essential for long-term success.
Join Us on Our AI Journey
The progression of AI automation in healthcare is a transformative journey that promises to revolutionize the industry.
From automating routine tasks to enabling sophisticated decision-making and achieving interoperability, AI is poised to make healthcare more efficient, accurate, and patient-centered.
If you believe your health system could benefit from digital self-triage, powered by integrated AI technology with clinical validation, let's chat.